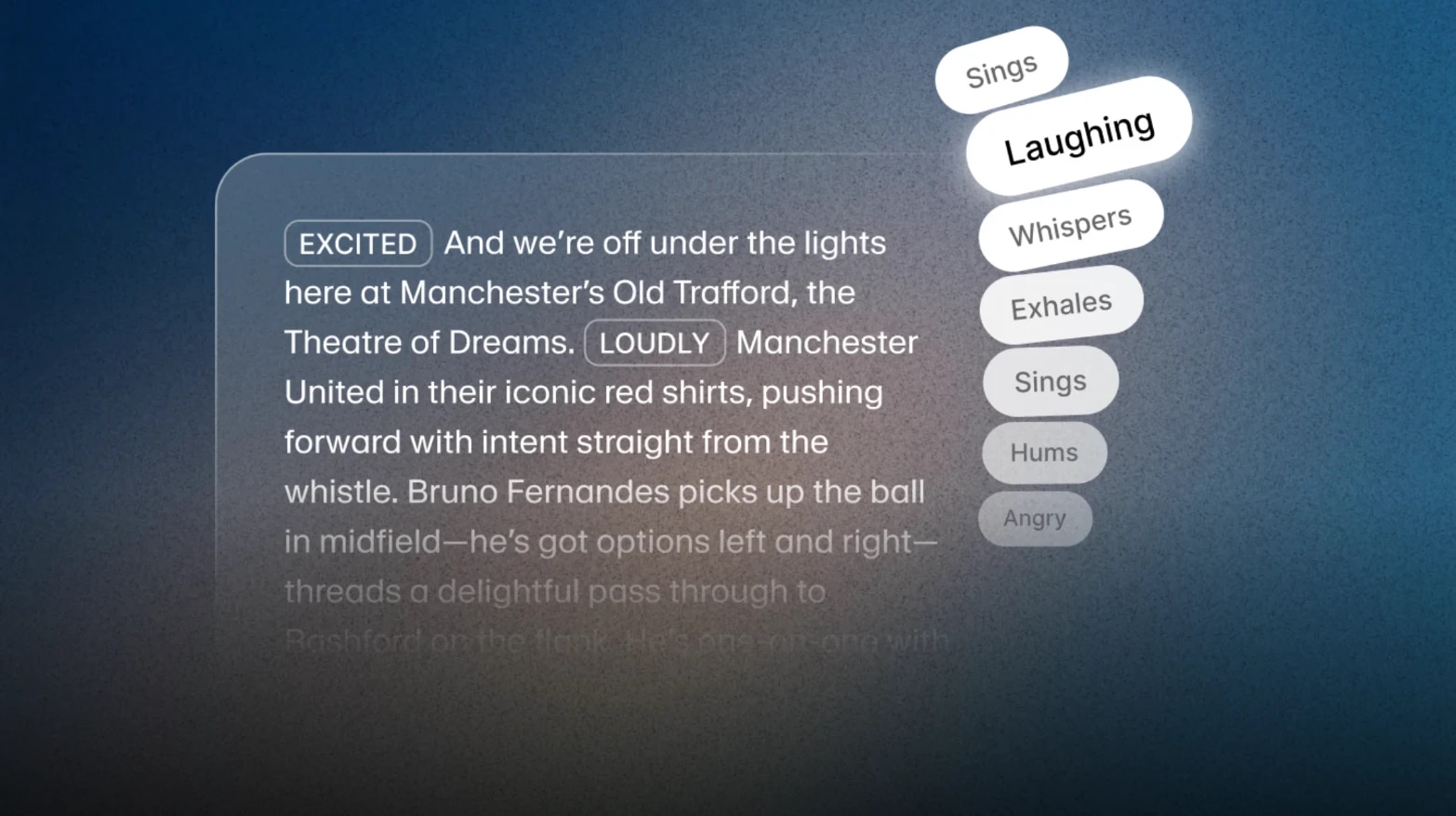
Eleven v3 Audio Tags: Emulating accents with precision
Seamlessly switch accents mid-sentence with Eleven v3 Audio Tags. Emulate American, British, French, and more for dynamic, culturally rich AI speech.
Introducing Eleven v3 (alpha)
Try v3In our last entry, we previewed a few long-form samples generated by our speech synthesis tool and we gave a brief overview of how our model’s unique design allows it to produce speech that’s well-paced and non-robotic. Today we’re going to show you that it’s also more emotionally rich and more context-aware than any other. This, in turn, makes it not only highly engaging to listen to but also well-suited for applications ranging from voicing books and video games to advertising.
Both our model's strengths - fluency and proper intonation - come from a wealth of training data it has seen (over 500k hours!), but really the central factor is how it learns from this data, which is down to the way it’s built. At the most basic level, it's made to understand the emotions contained in writing and to decide whether the speaker should sound happy, angry, sad or neutral. Consider a few examples:
All differences in intonation and mood come purely from text - nothing else influenced the output. Punctuation and the meaning of words play a leading role in deciding how to deliver a particular sentence but notice also how when the speaker is happy with victory, the model convincingly produces sounds which are not part of regular speech, like laughter (we will release a compilation of the different laughs our AI is capable of shortly!). Likewise, it appropriately exaggerates the reaction when the speaker is amused by something hilarious - it’s ‘sooooo funny’.
But knowing the meaning of individual words is not enough. Our model is equally sensitive to the wider situation surrounding each utterance - it assesses whether something makes sense by how it ties to preceding and succeeding text. This zoomed-out perspective allows it to intonate longer fragments properly by overlaying a particular train of thought stretching multiple sentences with a unifying emotional pattern, as shown in our previous entry containing lengthier content. But it also helps it avoid making logical mistakes. For example, some words are written in the same way but have different meaning e.g. ‘read’ in the present and past tenses or ‘minute’ meaning a unit of time or something small. Deciding which one is appropriate when depends on the context:
Because we design our platform to meet long-form content demands, we also need our model to understand that symbols and abbreviations and certain conventions which are common in writing should be pronounced in a particular way or not be pronounced literally. For example, the model needs to know that FBI, TNT and ATM are pronounced differently to UNESCO or NASA. Similarly, $3tr is perfectly fine in writing but when read aloud, it needs to become ‘three trillion dollars’.
Recognizing these subtle distinctions is crucial since our goal is to minimize the need for human intervention in the generation process. After all, we don't promote our tool's ability to generate an audiobook in minutes in order for someone to have to listen through the whole audio to then re-write the entire text. Nonetheless, even though we continuously update our model's rules on pronunciation, it's always possible that something will confuse it. To this end, we're now developing a system for flagging uncertainty which will allow users to instantly see which bits of text the model found problematic and let them teach it on how they should be said.
All the capabilities we've shown are steps on the way to making our software the most versatile AI voicing tool.
News publishers have already found that increasing their audio presence is a great way of retaining subscribers. The great benefit of embedding each article with its audio reading is that people can listen while doing something else. Those publishers who do so often use voice actors which is expensive and not all articles get covered. Or they use their own reporters to read stories which is time-consuming, meaning also expensive. Those who use synthetic speech to voice their content save money but pay another price by compromising on quality. Now, with Eleven Labs, there's no need to compromise and you can have the best of both worlds.
Or imagine generating audiobooks with distinct, emotionally compelling voiceover for all characters, within minutes. Not only does this present new ways of engaging with books but also greatly eases access for people with learning difficulties.
Just think of the possibilities now open to video game developers who no longer need to consider whether a particular character is important enough to justify the otherwise considerable cost of voicing them with real actors. All NPCs can now have their own voices and personalities.
Advertising agencies and producers can now freely experiment and adjust voiceovers to suit the tone of any campaign - whether it's for a sports TV channel or for a luxury watch brand. Any actor's voice can be licensed for cloning so that changes can be applied instantly and without the actor physically present. Or if they decide to go with a fully synthetic voice, advertisers also don't have to worry about paying buyouts for voice rights.
Virtual assistants can become more lifelike both because voice cloning allows them to speak with a voice that's familiar to a particular user and also because this newfound depth of delivery would make them more natural to interact with.
Go here to sign up for our beta platform and try it out for yourself. We're constantly making improvements and all user feedback is very valuable for us at this early stage. Enjoy!
Seamlessly switch accents mid-sentence with Eleven v3 Audio Tags. Emulate American, British, French, and more for dynamic, culturally rich AI speech.
Fine-grained control over timing, rhythm, and emphasis with Eleven v3 Audio Tags. Transform flat delivery into dynamic, performative content.